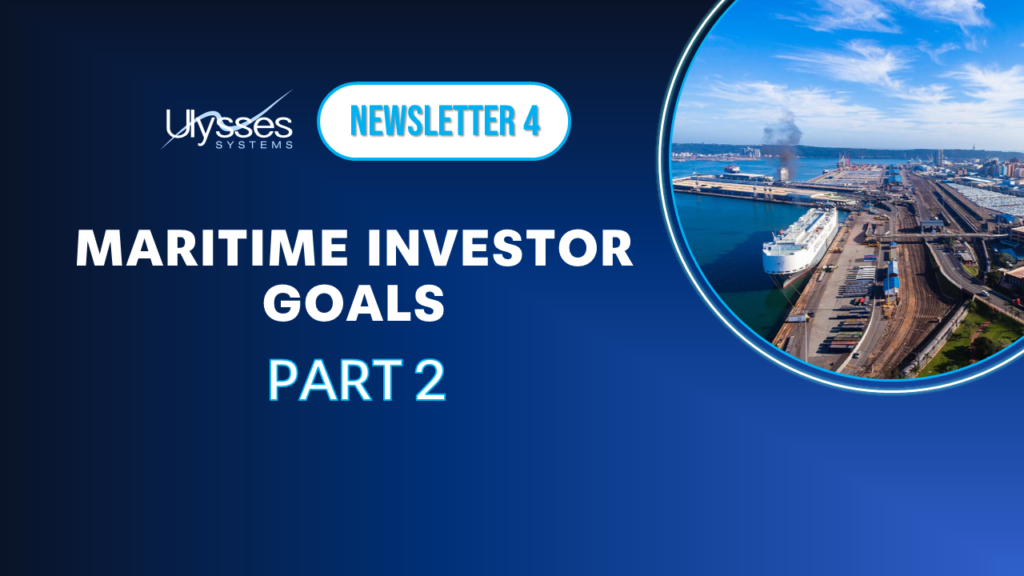
Maritime Investor Goals
The insuperable adeptness of human learning and the associative power of generative AI
The purpose of this article, Maritime Investor Goals, is to discuss the essentials of creating training material for a machine that searches by relevance based on modelled knowledge structures. The article consists of two parts:
Part 1: The essentials of creating training material for a machine that searches by relevance based on modelled knowledge structures. This sections emphasizes why it is necessary to create training material that differs from pattern recognition in which generative AI excels. And the section will end by describing what the enterprise search essentials for maritime investor goals are.
The adeptness of human learning and the associative power of generative AI
In Part 2 of Marine Investor Goals, we discuss the insuperable adeptness of human learning and the associative power of generative AI. Essentially, the section goes into more depth describing human aptitude in learning and understanding the world. And this is contrasted with the associative strength of generative AI. Our hope is to convince our friends and readers that we need more discussions on training machines. And to suggest technology solutions that can organize enterprise knowledge around the relevant maritime investor goals.
Going into greater depth to describe the human aptitude to learn and understand means highlighting the qualitative difference between goal-based human intelligence and the associative agility of AI. A more simple way to say this is: reasons why humans are intelligent, and AI is not. Our intention is not to denigrate the power of machine learning, which is, in fact, a human accomplishment. On the contrary! This analysis aims to resolve possible misconceptions and moderate the expectations maritime investors have of generative AI. And to point towards a technology that organizes goal-based knowledge structures around maritime investor goals.
Associations
In associations, no doubt, machine learning performs better than humans do.
And the more the training material, the more open generative AI is to new text and capable of accurately retrieving related material. This is similar to humans remembering associated subjects to the one at hand. Only that generative AI surpasses humans in this respect by many orders of magnitude.
But human ability to find associations is not a pillar of human intelligence. Human intelligence is based on making plans based on experience, with the intention of achieving goals and gaining more experience. In turn, this promotes better understanding of how the world works to assist or impede the goals.
So the question is how we can leverage this superior associative capability without confusing it with human-like intelligence.
Understanding the world
There is more to the way humans learn and thereby build an understanding of the world. So, despite the prominence of goals in human learning, setting a goal is only the contributing trigger to understanding. Essentially, it is in our efforts to achieve our goals that we learn and gain understanding. And this happens when we interact with the world which includes others like us. And we start early and some of the learning takes place without teaching or training!
In fact, from infancy we learn things about the world that we are not actually taught. For example, we recognise the apparent goals of other people that may conflict with ours. We learn the workings of the world from a social and physical standpoint. And our foundational learning competencies, complemented by experiences, lead to critical thinking.
Finally, we also learn about things we are interested in. In this case, in order to learn, we not only involve our minds but also our emotions. Voluntarily.
All of which a machine cannot do.
Learning in intrinsic ways
There are still more things a machine cannot learn that we do in intrinsic ways. Machines cannot learn on the go like we can; we learn by doing, and as we consider the obstacles, we apply the solutions to arrive at our goal.
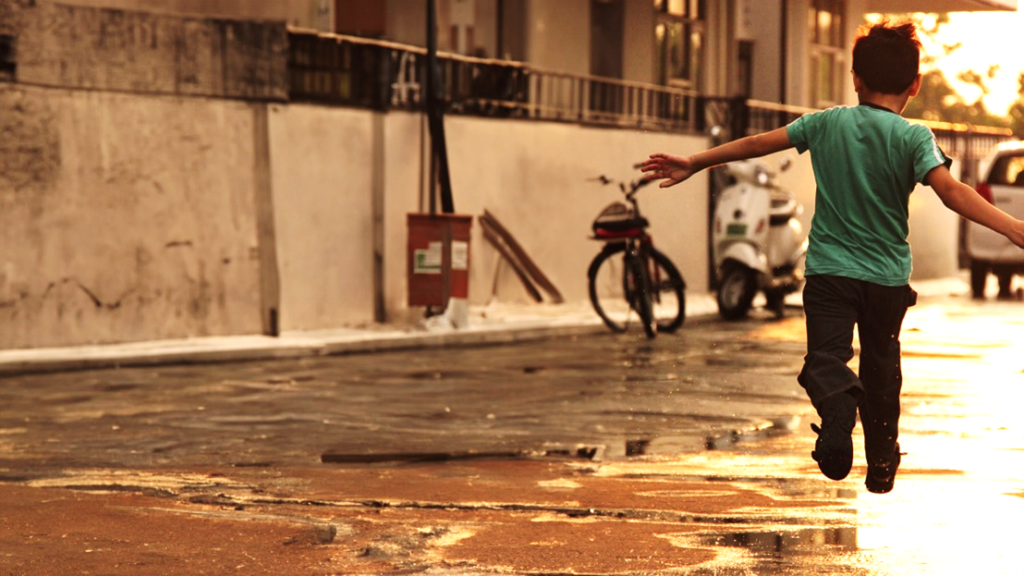
We also learn by reflection, which is about comparing the experience in one case with the experience in another. And doing so leads us to draw conclusions and make predictions based on similarity of cases.
Our astonishing and rich capacity to learn relies on an innate grouping of goal-based experiences in relevant groups and managing the new features that constitute the learning aspect. And added to this is the capability we have of retrieving them from memory when we need them.
We also learn by reflection, which is about comparing the experience in one case with the experience in another. And doing so leads us to draw conclusions and make predictions based on similarity of cases.
Our astonishing and rich capacity to learn relies on an innate grouping of goal-based experiences in relevant groups and managing the new features that constitute the learning aspect. And added to this is the capability we have of retrieving them from memory when we need them.
Facts
Other learning methods are founded on this innate learning capacity, including learning facts. Facts help reinforce goal/process scenarios if they are provided at the time of need. This is important because we don’t need to keep in mind facts irrelevant to what we are doing in the present. For instance, when we prepare a plan, we remember specific facts useful for achieving our plan, not all the facts we have ever learnt. In fact, this is an innate separation of concerns mechanism we have, which a machine can emulate providing it has a goal model.
Knowing what to expect
The learning methods that we have used throughout the journey of humanity provide a fundamental expectation of how the world works and a foundational framework for knowing what to expect.
We don’t entirely know the framework that maintains our learning adeptness. Nevertheless, we can’t but observe that humans are experts in how one process affects another. And at how the world of other people and entitles, for example cultural, legal and social, plus the physical world becomes relevant to our activities.
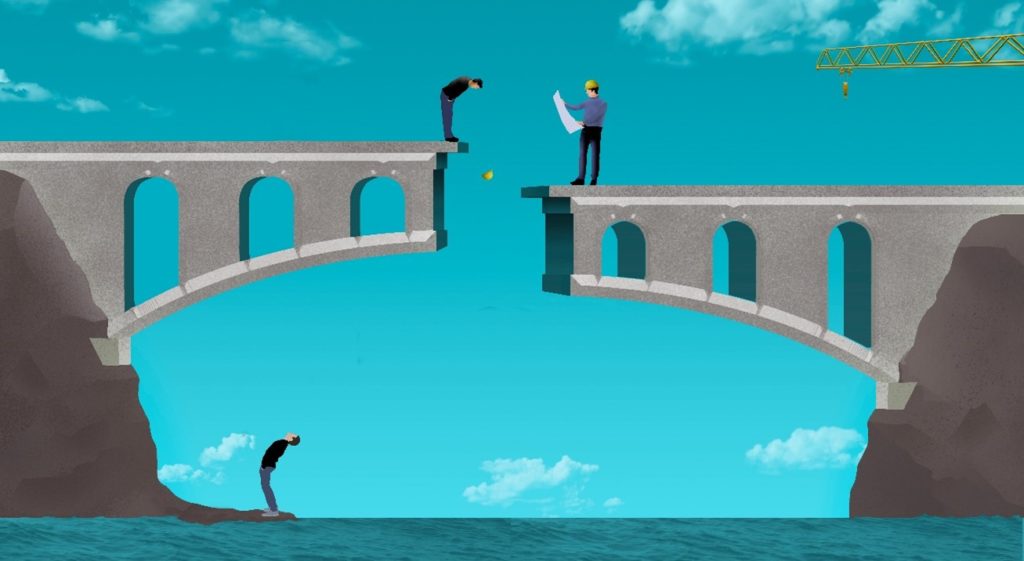
We can appreciate, then, why machine learning and generative AI is not making big steps in true understanding.
Pattern recognition
But it is making big steps in pattern recognition, and this helps in areas where pattern recognition is needed on a grand scale. It is an adeptness humans don’t have at scale, but neither is it pivotal for most enterprises.
Web search
But for web search pattern recognition at scale is a quantum leap. Essentially, because the web is vast, and correlation is the only scalable way to relate things. It is the key to accurate retrievals, so, sophisticated methods to correlate is progress.
Enterprise Search
Pivotal for the enterprise is a search tool with the ability to detect impending risk in very large systems. We saw that humans have a foundational knowledge of how one process affects another and what to expect. And they do this well. But computers can also do so in a scalable way, even across vast numbers of related enterprise processes.
And a machine that reads text could also highlight missing processes within a real world goal model. Domain experts can then supervise the expansion of the model by comparing it to other similar models using in-built facilities.
So, for a machine that searches by relevance based on a modelled knowledge structure, comparing and expanding the model can be an added capability.
Competence of a machine in comparing and adjusting
We can therefore conclude that competence of a machine in comparing and adjusting its model of the world, in order to encompass more of the real world, is closer to human learning than correlating and querying texts. Also, such a machine aligns cause and effect propagations, organizes data, information exchanges and stories around enterprise goals, searching and retrieving content with incomparable relevancy.
Closing words
We sincerely hope you found value reading ‘Enterprise Search Essentials For Maritime Investor Goals’ the third article in a series on generative AI.
In a similar vein, we feel you will find the article “Future of AI” by Dimitris Lyras, relevant.
You may also wish to refer to the prequel to the current article ‘Automated Learning for Computers Useful for the Marine Vertical’
And if you enjoyed the newsletter, please help us by subscribing and sharing. Our goal with our newsletters is to enter into discussion with our readers on topics discussing hot technology subjects in the context of the maritime industry’s painpoints.
Ulysses Systems is a Maritime Software specialist. Its award-winning Task Assistant® Software enables office and seagoing personnel to work intuitively and efficiently with minimal training and just-in-time information. Managers should expect a fast return on total software lifecycle cost thanks to mature process optimization, bridging of information gaps and refined integration technologies. Currently Ulysses Systems is pioneering fast development of new annexes to existing software, including monitoring underlying systems for cybersecurity compliance.
References
Stephen Wolfram, ‘What is ChatGPT doing and why does it work’